The momentum behind autonomous vehicles has the power to revolutionise the insurance industry, but it could be decades before regulators allow vehicles to be built without manual controls and the fact remains that we are still far from a driverless reality. However, some of the technologies integral to the autonomous future are already helping to shape better, more self-aware drivers, today.
In the halfway-house between the old world and a safer, self-driving future, telematics technology is having a particularly notable impact of the way we drive. In Octo's case, dynamic machine learning algorithms supported by the world's largest insurance telematics database are revolutionising not only the economics, but also the behavioural science of driving.
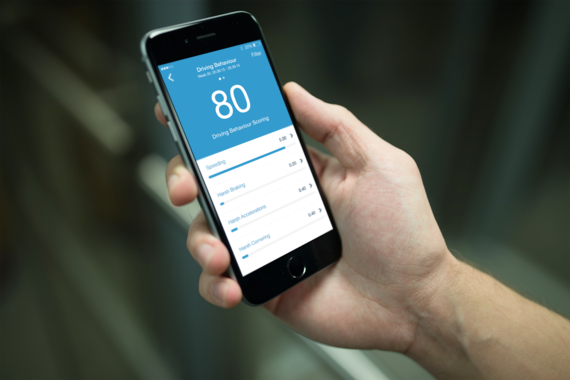
The change, of course, does not come from the numbers themselves, but from making sense of the data extracted from connected cars. The data we once allowed to go uncaptured now can be feed into algorithms, which provides insights into driver behaviour, and in turn, allows both drivers and insurers to take action. Just like a health app on a smartphone, getting instant feedback on your driving can provide powerful nudges to change behaviour - for example, to drive more carefully and economically.
This in turn has a direct influence on something that data capture finally makes truly measurable: risk. Currently, insurers have to price their policies based on very rough and ready assumptions; for example, a driver's age or the model of car they own, regardless of their actual driving behaviour. However, by harnessing driver data and applying sophisticated data analytics, telematics companies can close the feedback loop to improve driver safety and give insurers the insights they need to forecast individual risk, reducing connected users' insurance premiums by up to 30%.
More broadly, connected cars are a particularly good example of man + smart machine - especially in demonstrating the power for technology to promote positive behavioural changes. Telematics contributes to promoting positive behavioural change around people engaging in a constructive manner with smart algorithms and machines in pursuing goals - in this case becoming better and safer drivers.
This social engineering potential of telematics is so under-appreciated. It doesn't just produce lower premiums and safer driving. It actually engenders behavioural change as part of the broader socio-economic transition from humans vs machines to humans + machines. With machines coaching our decision-making, we become better and safer drivers, and risks are better managed. Similarly, we are coaching and teaching the constantly evolving smart algorithms that sit behind connected cars about managing risks and social behaviours.
It is important to consider the 'panopticon' effect and the reality that, as we are predicting human behaviour, we are also shaping it. This is one of the few examples in action of both the opportunities and risks of predictive analytics tipping over into prescriptive analytics.
The predictive analytics of telematics is, in large part, helping to achieve a prescriptive vision of safer and more affordable travel. Predictive analytics is also contributing to promotion of positive behavioural change around people engaging in a constructive manner with smart algorithms and machines in pursuing goals - in this case becoming better and safer drivers.
Thanks to the telematics and the power of the Algorithm Economy, driving is at the forefront of the machine learning transition.