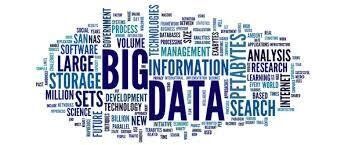
A yottabyte equals 1 trillion terabytes (the largest data metric that most commonly used today). That's not just big data, it's really big data, and it's clearly the direction that things are heading in; particularly given the prevalence of today's "keep everything" mantra. It's this thinking that when combined with the equally pernicious belief that "storage is cheap" has brought legal firms, private and public companies and government agencies alike to the data deluge precipice, where even the possibility of big data can't provide salvation.
In order to create a more sustainable data management future, several big data myths need to be debunked.
Myth #1 - All Data is Valuable
Under the first big data myth, many tend to believe that the 3 V's of big data (Volume, Velocity, Variety) are all that matter. The thinking goes that even if we can't make sense of data right now, in the future, big data applications will continue to advance so that historical data troves can be mined for useful nuggets.
One use case is at least conceptually compelling. Assume, for example, that a company has 10 years of unexamined data relating to a particular area of its business. Hypothetically, at some point in the future, it is then able to leverage big data analytics to examine this historical information in an effort to predict future customer trends. The historical data could be used to validate that the prediction engine is accurate, by looking at data from years one-nine to predict what will happen in year ten (which has already occurred). This way, the argument goes, the prediction capabilities could then be validated by the historical data before they're applied to the future.
The fault in this logic is the failure to realise that all data isn't created equally. Data Value (as an additional V) is a critical component in the big data equation. Not only is the value of data subjective, to the analytical task at hand, but often it has a definitive shelf life. For example, customer sentiment data (how a client feels about a given product or solution) may be very short lived. Consider whether a six-month-old satisfaction rating regarding a dinner at an upscale restaurant has any value after the restaurant has profoundly changed its menu. It might be to conceivably show how the new cuisine is faring, but then think if this information still has value after another twelve months.
This illustrates how data value decreases over time, but what about information that has no information value to begin with. In a recent survey by the Compliance, Governance and Oversight Council, it was revealed that 69% of an average company's data had no legal, regulatory, legal hold or other business value. This mix contains duplicate (and near duplicate) data, employee's personal information and other corporate noise that's only tangentially related to the core business at task.
The bottom line is that retaining more of this valueless data won't yield better results even with the advent of big data initiatives. In fact, the opposite is true.
Myth #2 - With Big Data the More Information the Better
Last October The Rand Corporation revealed in its Data Governance Survey that the participants "expected data growth in the next year "between 26% and 50%, with several participants indicating they expect data growth of more than 200% over the next year." The median amount of data stored by survey respondents was between 20TB to 50TB, with a shocking 22% in the petabyte range.
While these data explosion headlines have largely become numbing, the fact is that more information is not inherently better (particularly if it doesn't have value, per above). What gets fewer headlines is the "signal to noise" aspect of data management run without limits. Probably best summarised in Nate Silver's The Signal and the Noise: Why So Many Predictions Fail -- but Some Don't is the notion that extraneous data noise has deleterious consequences. The book's premise comes from the electrical engineering field, where a signal is something that conveys information, while noise is an unwanted/irrelevant addition to the signal. The problem occurs (and is particularly acute regarding to big data analytics) when it's nearly impossible to tell which is which: valuable "signal" or distracting "noise."
This signal/noise issue is the final straw that makes governance truly a near term corporate imperative. Even assuming a company is willing to roll the dice on expanding eDiscovery costs, botched regulatory compliance and periodic privacy breaches (perhaps under the belief that those elements don't grow the business), workers must be able to find the right information at the right time to do their jobs. So yes, storage is relatively cheap, but the ramifications of a "store everything forever" can be quite expensive.
Myth #3 - Big Data Opportunities Come with No Costs
Despite the foregoing, it's clear that big data can have value to an organisation - assuming the right data is harnessed at the right time. But even then, there is the flip side of the coin - i.e., how much does it cost to keep around terabytes of data that aren't yet being harnessed for big data analytics?
This is where the concept of information governance (IG) comes to the forefront. IG can be defined as:
"A cross-departmental framework consisting of the policies, procedures and technologies designed to optimise the value of information while simultaneously managing the risks and controlling the associated costs, which requires the coordination of eDiscovery, records management and privacy/security disciplines."
Last year's AIIM study, Information governance - records, risks and retention in the litigation age, highlights the fact that senior management is ignoring the risks. The study found that 31% admitted their inferior electronic records keeping is causing problems with regulators and auditors, while 14% said they were incurring fines or bad publicity due to bad handling of information.
Here, the dark side of big data is often not counterbalanced against the potential value. eDiscovery is perhaps the easiest and most tangible way to illustrate the risks and costs of keeping data. In a recent survey, the Rand Corporation determined that it costs on average, $18,000 (roughly £10,750) to review a single gigabyte of content for eDiscovery purposes. Given that even medium sized eDiscovery cases can run in the hundreds of gigabytes, it's easy to see how this type of data, just by lying around, can and does have associated costs.
At the end of the day, good data hygiene means that organisations should keep what's valuable and purge what's not. This unassailable fact doesn't really change despite the emergence of big data. Savvy counsel is advised to watch out for these and other myths in this "keep it forever" era.